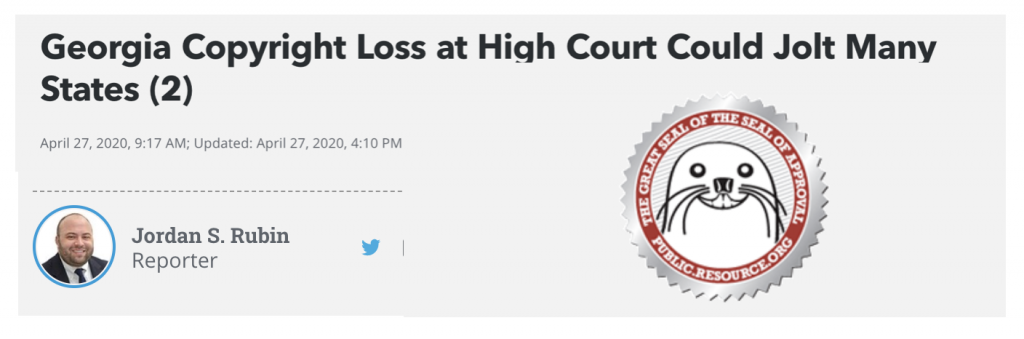
Very happy to see this favorable ruling! Coverage in Bloomberg Law. I was one of 36 signatories to one of the Amicus Briefs in the case.
Very happy to see this favorable ruling! Coverage in Bloomberg Law. I was one of 36 signatories to one of the Amicus Briefs in the case.
Today is the day that SCOTUS considers Georgia v. Public.Resource.Org, Inc. (18-1150) — https://www.oyez.org/cases/2019/18-1150
I am one of the 36 Scholars who have published Computational Law Papers and who are signatories to an Amicus Brief in State of Georgia vs Public.Resource.Org (case in front of the Supreme Court of the United States this term). The Link to the Amicus Brief is here. The Link to the Full List of Signatories is here.
This is a very interesting Net Sci + Law Paper !
Here is Version 2.01 of the Law on the Market Paper —
From the Abstract: What happens when the Supreme Court of the United States decides a case impacting one or more publicly-traded firms? While many have observed anecdotal evidence linking decisions or oral arguments to abnormal stock returns, few have rigorously or systematically investigated the behavior of equities around Supreme Court actions. In this research, we present the first comprehensive, longitudinal study on the topic, spanning over 15 years and hundreds of cases and firms. Using both intra- and interday data around decisions and oral arguments, we evaluate the frequency and magnitude of statistically-significant abnormal return events after Supreme Court action. On a per-term basis, we find 5.3 cases and 7.8 stocks that exhibit abnormal returns after decision. In total, across the cases we examined, we find 79 out of the 211 cases (37%) exhibit an average abnormal return of 4.4% over a two-session window with an average |t|-statistic of 2.9. Finally, we observe that abnormal returns following Supreme Court decisions materialize over the span of hours and days, not minutes, yielding strong implications for market efficiency in this context. While we cannot causally separate substantive legal impact from mere revision of beliefs, we do find strong evidence that there is indeed a “law on the market” effect as measured by the frequency of abnormal return events, and that these abnormal returns are not immediately incorporated into prices.
ABSTRACT: Do judicial decisions affect the securities markets in discernible and perhaps predictable ways? In other words, is there “law on the market” (LOTM)? This is a question that has been raised by commentators, but answered by very few in a systematic and financially rigorous manner. Using intraday data and a multiday event window, this large scale event study seeks to determine the existence, frequency and magnitude of equity market impacts flowing from Supreme Court decisions.
We demonstrate that, while certainly not present in every case, “law on the market” events are fairly common. Across all cases decided by the Supreme Court of the United States between the 1999-2013 terms, we identify 79 cases where the share price of one or more publicly traded company moved in direct response to a Supreme Court decision. In the aggregate, over fifteen years, Supreme Court decisions were responsible for more than 140 billion dollars in absolute changes in wealth. Our analysis not only contributes to our understanding of the political economy of judicial decision making, but also links to the broader set of research exploring the performance in financial markets using event study methods.
We conclude by exploring the informational efficiency of law as a market by highlighting the speed at which information from Supreme Court decisions is assimilated by the market. Relatively speaking, LOTM events have historically exhibited slow rates of information incorporation for affected securities. This implies a market ripe for arbitrage where an event-based trading strategy could be successful.
Available on SSRN and arXiv
Readers will probably observe that {Marshall+} is still a work in progress (for example – my colleague noted {Marshall+} believes that Justice Ginsburg would appear to be slightly more likely to vote to overturn the ACA than Justice Thomas). While this probably will not prove to be correct in King v. Burwell, our method is rigorously backtested and designed to minimize errors across all predictions (not just in this specific case). This optimization question is tricky for the model and it will be the source of future model improvements. I have preached the whole mantra Humans + Machines > Humans or Machines and this problem is a good example. The problem with exclusive reliance upon human experts is they have cognitive biases, info processing issues, etc. The problem with models is that they generate errors that humans would not.
Anyway, the good thing about having a base model such as {Marshall+} is that we can begin to incorporate a range of additional information in an effort to create a {Marshall++} and beyond. And on that front there is more to come …
Here is an introductory slide deck from “Legal Analytics” which is a course that Mike Bommarito and I are teaching this semester. Relevant legal applications include predictive coding in e-discovery (i.e. classification), early case assessment and overall case prediction, pricing and staff forecasting, prediction of judicial behavior, etc.
As I have written in my recent article in Emory Law Journal – we are moving into an era of data driven law practice. This course is a direct response to demands from relevant industry stakeholders. For a large number of prediction tasks … humans + machines > humans or machines working alone.
We believe this is the first ever Machine Learning Course offered to law students and it our goal to help develop the first wave of human capital trained to thrive as this this new data driven era takes hold. Richard Susskind likes to highlight this famous quote from Wayne Gretzky … “A good hockey player plays where the puck is. A great hockey player plays where the puck is going to be.”
Today I am excited to announce that LexPredict has now launched the all new FantasySCOTUS under the direction of Michael J. Bommarito II, Daniel Martin Katz and Josh Blackman.
FantasySCOTUS is the leading Supreme Court Fantasy League. Thousands of attorneys, law students, and other avid Supreme Court followers make predictions about cases before the Supreme Court. Participation is FREE and Supreme Court geeks can win cash prizes up to $10,000 (many other prizes as well — thanks to the generous support of Thomson Reuters).
We hope to launch additional functionality soon but we are now live and ready to accept your predictions for the 2014-2015 Supreme Court Term!
Abstract: “Building upon developments in theoretical and applied machine learning, as well as the efforts of various scholars including Guimera and Sales-Pardo (2011), Ruger et al. (2004), and Martin et al. (2004), we construct a model designed to predict the voting behavior of the Supreme Court of the United States. Using the extremely randomized tree method first proposed in Geurts, et al. (2006), a method similar to the random forest approach developed in Breiman (2001), as well as novel feature engineering, we predict more than sixty years of decisions by the Supreme Court of the United States (1953-2013). Using only data available prior to the date of decision, our model correctly identifies 69.7% of the Court’s overall affirm and reverse decisions and correctly forecasts 70.9% of the votes of individual justices across 7,700 cases and more than 68,000 justice votes. Our performance is consistent with the general level of prediction offered by prior scholars. However, our model is distinctive as it is the first robust, generalized, and fully predictive model of Supreme Court voting behavior offered to date. Our model predicts six decades of behavior of thirty Justices appointed by thirteen Presidents. With a more sound methodological foundation, our results represent a major advance for the science of quantitative legal prediction and portend a range of other potential applications, such as those described in Katz (2013).”
You can access the current draft of the paper via SSRN or via the physics arXiv. Full code is publicly available on Github. See also the LexPredict site. More on this to come soon …
From the Abstract: “This Article proposes a novel and provocative analysis of judicial opinions that are published without indicating individual authorship. Our approach provides an unbiased, quantitative, and computer scientific answer to a problem that has long plagued legal commentators. Our work uses natural language processing to predict authorship of judicial opinions that are unsigned or whose attribution is disputed. Using a dataset of Supreme Court opinions with known authorship, we identify key words and phrases that can, to a high degree of accuracy, predict authorship. Thus, our method makes accessible an important class of cases heretofore inaccessible. For illustrative purposes, we explain our process as applied to the Obamacare decision, in which the authorship of a joint dissent was subject to significant popular speculation. We conclude with a chart predicting the author of every unsigned per curiam opinion during the Roberts Court.” <HT: Josh Blackman>