Month: March 2010
United States Supreme Court’s New Website — Still Far Below the Mark
The United States Supreme Court has recently launched its newly redesigned website. Given the significant limitations associated with the prior website, this launch had been highly anticipated. Indeed, many had hoped for a website that would reflect well upon the Court. While we applaud the decision to move away from the prior design, a review of the Court’s new website reveals a product which still falls far below the mark.
One of the very disappointing aspects of the new interface is that it appears to have been created with little regard for the overtures offered by folks such as the Sunlight Foundation. Specifically, in an effort to improve the experience of end users, the Sunlight Foundation offered a very constructive mockup redesign for the website. Unfortunately, the current redesign does not reflect most of their ideas.
For those interested in additional thoughts on this matter, the good folks over at Law Librarian Blog offer a number of constructive suggestions for improvements. These include unlocking PDF documents by simultaneously making their content available in friendly formats such as XML / HTML. Anyway, we hope that this is merely one step in the direction of genuine improvement …. but given the current state of affairs … the path to a 21st century website for the United States Supreme Court looks to be quite long.
Visualizing a Subset of the Tax Code – Capital Gains & Losses at Full Depth [Repost]
In our original post on Title 26, we displayed every subtitle of Title 26, A through K, down to the section level. We did not, however, display the elements of the code below the section level. For example, our prior visualization stops at §501 and does not distinguish between §501(c)(3) and §501(c)(2). This is a function of the size of Title 26, as the full labeled image is too large to render and distribute over the Internet. However, working with only a subset of Title 26 of the United States Code, we can provide a full depth zoomable visualization.
We’ve chosen to highlight – Subtitle A, Chapter 1, Subchapter P: Capital Gains and Losses as this section well represents the structural complexity of the code. Despite only displaying a single subchapter without any Treasury Regs or SEC guidance, there are over 1,711 citable elements in this visual. If you look closely you can see the labels for each and every piece of this subchapter, including §1221(b)(2)(A)(ii), which defines “hedging transactions” (e.g., swaps) for the purpose of the tax code: “to manage risk of interest rate or price changes or currency fluctuations with respect to borrowings made or to be made, or ordinary obligations incurred or to be incurred, by the taxpayer.” For details on the coloring of this visual, see our previous post visualizing the Bankruptcy Code 11 U.S.C located here.
H.R. 4872 Word Cloud
With the passage of H.R. 4872 in the House last night, our previous research on the relative size of H.R. 3962 has been in high demand. While we are not yet prepared to run similar calculations for H.R. 4872, at first glance we can say – as passed, H.R. 4872 is marginally longer H.R. 3200 and nearly 20% longer than H.R. 3962.
We have created the word cloud below … it is mainly just for fun. However, one interesting thing that jumps out from the cloud is the prevalence of the word “secretary.” Indeed, this may indicate the legislation provides a non-trivial amount of discretion to the relevant various administrative agencies.
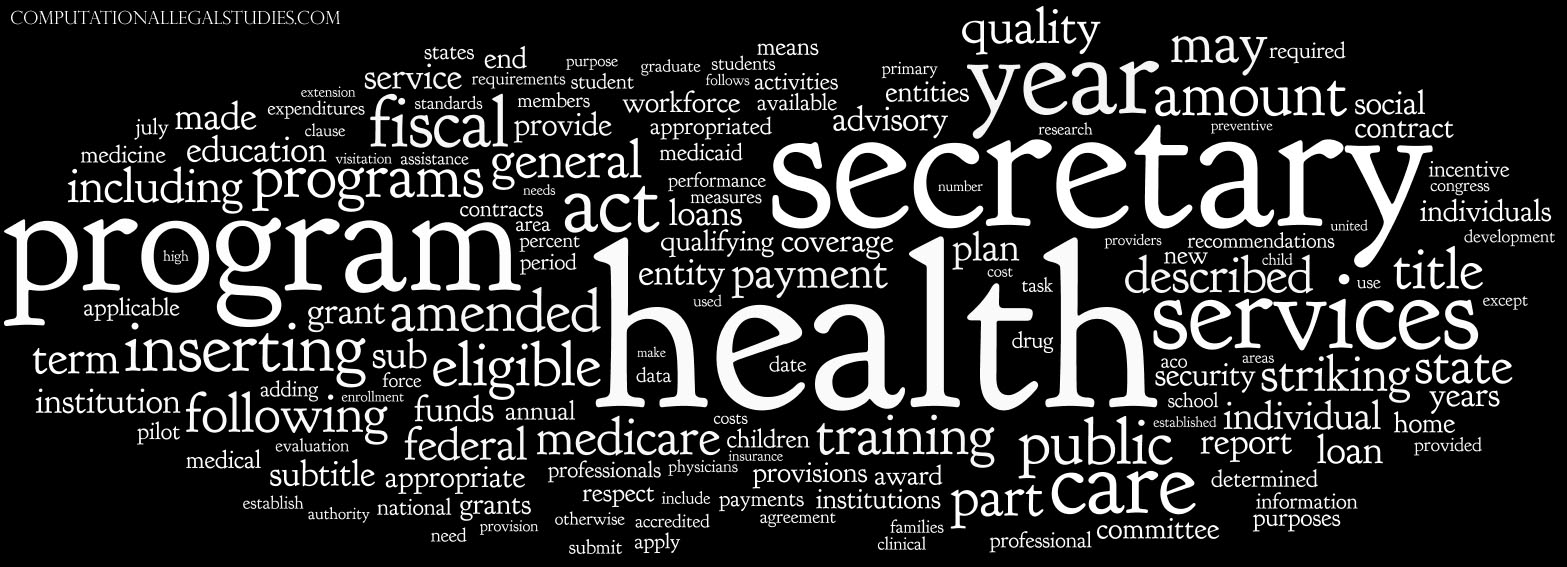
Detrending Career Statistics in Professional Baseball: Accounting for the Steroids Era and Beyond
From the abstract: “There is a long standing debate over how to objectively compare the career achievements of professional athletes from different historical eras. Developing an objective approach will be of particular importance over the next decade as Major League Baseball (MLB) players from the “steroids era” become eligible for Hall of Fame induction. Here we address this issue, as well as the general problem of comparing statistics from distinct eras, by detrending the seasonal statistics of professional baseball players. We detrend player statistics by normalizing achievements to seasonal averages, which accounts for changes in relative player ability resulting from both exogenous and endogenous factors, such as talent dilution from expansion, equipment and training improvements, as well as performance enhancing drugs (PED). In this paper we compare the probability density function (pdf) of detrended career statistics to the pdf of raw career statistics for five statistical categories — hits (H), home runs (HR), runs batted in (RBI), wins (W) and strikeouts (K) — over the 90-year period 1920-2009. We find that the functional form of these pdfs are stationary under detrending. This stationarity implies that the statistical regularity observed in the right-skewed distributions for longevity and success in professional baseball arises from both the wide range of intrinsic talent among athletes and the underlying nature of competition. Using this simple detrending technique, we examine the top 50 all-time careers for H, HR, RBI, W and K. We fit the pdfs for career success by the Gamma distribution in order to calculate objective benchmarks based on extreme statistics which can be used for the identification of extraordinary careers.”
Happy Birthday to Computational Legal Studies
On March 17, 2009 we offered our first post here at the Computational Legal Studies Blog. It has been an exciting and fun year. Here are some of the highlights!
New Paper Available on SSRN: A Profitable Trading and Risk Management Strategy Despite Transaction Cost
Readers might be interested in an article that A. Duran and I have coming out in Quantitative Finance this year entitled A Profitable Trading and Risk Management Strategy Despite Transaction Cost. In the article, we develop a strategy which outperforms the “market” in rigorous out-of-sample testing. We’ve made sure to check the robustness of the results by performing Monte Carlo simulations on both the S&P 500 and Russell 2000 while varying the subsets of stocks and time periods used in the simulation.
The strategy is interesting in that it is based on behavioral patterns. Unlike many other algorithmic trading models, our strategy is modeled after a human trader with quarterly memory who categorizes the market return distribution and market risk into low, medium, and high categories. Technically, it accomplishes this by non-parametrically categorizing windowed estimates of the first four moments of the return distribution and the normalized leading eigenvalue of the windowed correlation matrix. Based on the assessment of these low/medium/high categories and past experience in similar states, the strategy then decides whether to invest in the market index, invest in the risk-free asset, or short the market. The strategy soundly outperforms the market index in multiple markets over random windows and on random subsets of stocks.
While you’re waiting for its publication in Quantitative Finance, you might check out a copy over at SSRN. Here’s the abstract and a figure below comparing the log-return of our strategy with the market over one realization:
We present a new profitable trading and risk management strategy with transaction cost for an adaptive equally weighted portfolio. Moreover, we implement a rule-based expert system for the daily financial decision making process by using the power of spectral analysis. We use several key components such as principal component analysis, partitioning, memory in stock markets, percentile for relative standing, the first four normalized central moments, learning algorithm, switching among several investments positions consisting of short stock market, long stock market and money market with real risk-free rates. We find that it is possible to beat the proxy for equity market without short selling for S&P 500-listed 168 stocks during the 1998-2008 period and Russell 2000-listed 213 stocks during the 1995-2007 period. Our Monte Carlo simulation over both the various set of stocks and the interval of time confirms our findings.
160,000 Hours of C-Span Coverage at Your Finger Tips
As reported in the NY Times … roughly 160,000 hours of C-SPAN coverage is going live for your consumption. Yet another example that the Era of Big Data is upon us!
The Dissemination of Culture — Axelrod (1997) Model — Now Available on Netlogo’s Community Models Page
Robert Axelrod’s 1997 Culture Model is a complex systems classic. Several versions of the model are available including one in Repast J. Perhaps the most user friendly version has recently been posted to Netlogo’s “community models” page. Those interested in experimenting with this Netlogo version of the model can click on the image above (provided you have Java 4.1 or higher installed).
For those not previously familiar with the model … Figure 1 from the article is featured to the left and demonstrates a model run through 80,000 events. Those results are generated in the following manner:
“Patches are assigned a list of num-features integers which can each take on one of num-traits values. Each tag is called a feature, while it’s value is called the trait. The links in the view represent walls between patches where solid black walls mean there is no cultural similarity, and white walls mean the neighbors have the same culture.
The order of actions is as follows:
1) At random, pick a site to be active, and pick one of it’s neighbors
2) With probability equal to their cultural similarity, these sites interact. The active site replaces one of the features on which they differ (if any) with the corresponding trait of the neighbor.”
Those looking for the original article … here is the both the citation and a link: Robert Axelrod, The Dissemination of Culture: A Model with Local Convergence and Global Polarization, J. Conflict Res, 41, 203 (1997).
In the years following its release, several important extensions or applications have been offered. These include contributions from scholars in a wide number of disciplines including applied math, political science, economics and physics. Indeed, while many more articles are available in outlets such as the arXiv … here is a subset for your consideration ….
Damon Centola, Juan Carlos González-Avella, Víctor M. Eguíluz & Maxi San Miguel, Homophily, Cultural Drift and the Co-Evolution of Cultural Groups, J. Conflict Res. 51, 905 (2007).
Konstantin Klemm, Victor M. Eguíluz, Raul Toral, Maxi San Miguel, Globalization, Polarization and Cultural Drift, J. Economic Dynamics & Control 29, 321 (2005).
Konstantin Klemm, Victor M. Eguíluz, Raul Toral & Maxi San Miguel, Role of Dimensionality in Axelrod’s Model for the Dissemination of Culture, Physica A 327, 1 (2003).
Gregory Todd Jones — Evolution of Complexity and “Rethinking Individuality” at TedX Atlanta
As a member of the Society for Evolutionary Analysis in Law (SEAL), I have had the oppurtunity to see a number of interesting presentations by Gregory Todd Jones. Gregory is a Faculty Research Fellow and Adjunct Professor of Law at the Georgia State University College of Law as well as Senior Director of Research and Principal Scientist at the Network for Collaborative Problem Solving. Of particular interest to readers of this blog, he is also the founding director of the Computational Laboratory for Complex Adaptive Systems at Georgia State Law School.
Above is a recent talk by Gregory at the TedX Atlanta in which he (1) assembles a model of sustainability based on collaboration and (2) discusses species behavior … from slugs to chimpanzees. If you are interested in learning more … Gregory has launched a really cool blog … Cooperation Science Blog … Check it out!
Is It Real, or Is It Randomized?: A Financial Turing Test
From the abstract … “We construct a financial “Turing test” to determine whether human subjects can differentiate between actual vs. randomized financial returns. The experiment consists of an online video-game where players are challenged to distinguish actual financial market returns from random temporal permutations of those returns. We find overwhelming statistical evidence (p-values no greater than 0.5%) that subjects can consistently distinguish between the two types of time series, thereby refuting the widespread belief that financial markets “look random.” A key feature of the experiment is that subjects are given immediate feedback regarding the validity of their choices, allowing them to learn and adapt. We suggest that such novel interfaces can harness human capabilities to process and extract information from financial data in ways that computers cannot.”