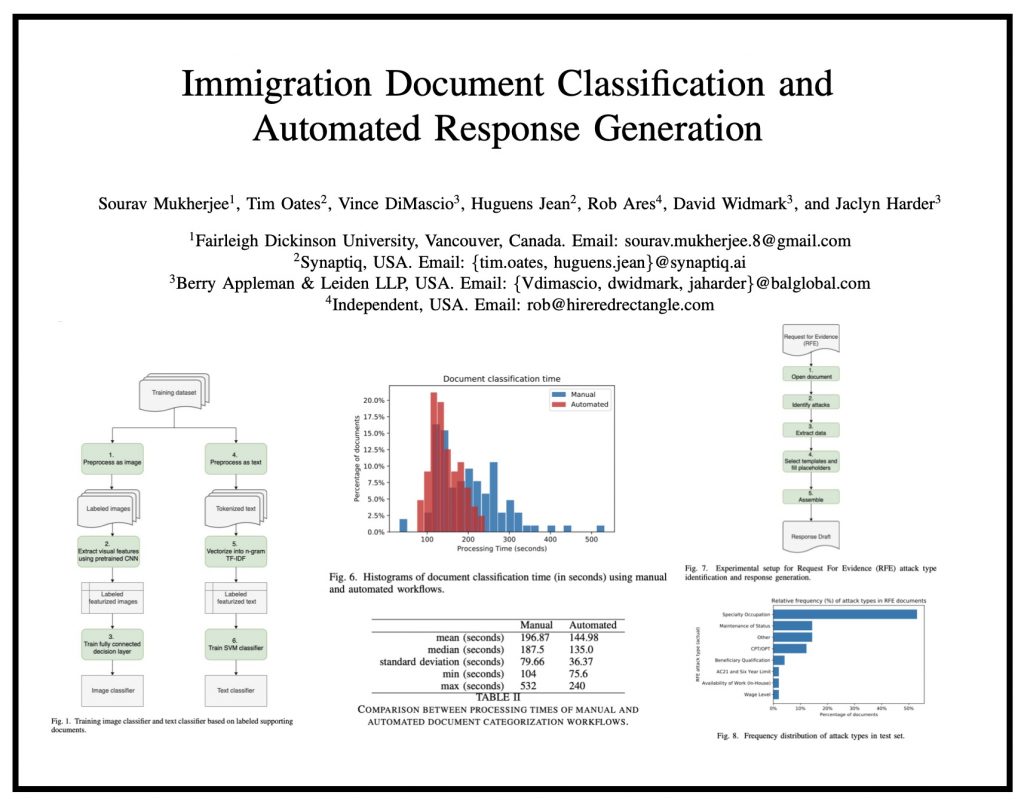
ABSTRACT: “In this paper, we consider the problem of organizing supporting documents vital to U.S. work visa petitions, as well as responding to Requests For Evidence (RFE) issued by the U.S.~Citizenship and Immigration Services (USCIS). Typically, both processes require a significant amount of repetitive manual effort. To reduce the burden of mechanical work, we apply machine learning methods to automate these processes, with humans in the loop to review and edit output for submission. In particular, we use an ensemble of image and text classifiers to categorize supporting documents. We also use a text classifier to automatically identify the types of evidence being requested in an RFE, and used the identified types in conjunction with response templates and extracted fields to assemble draft responses. Empirical results suggest that our approach achieves considerable accuracy while significantly reducing processing time.” Access Via arXiv — To Appear in ICDM 2020 workshop: MLLD-2020