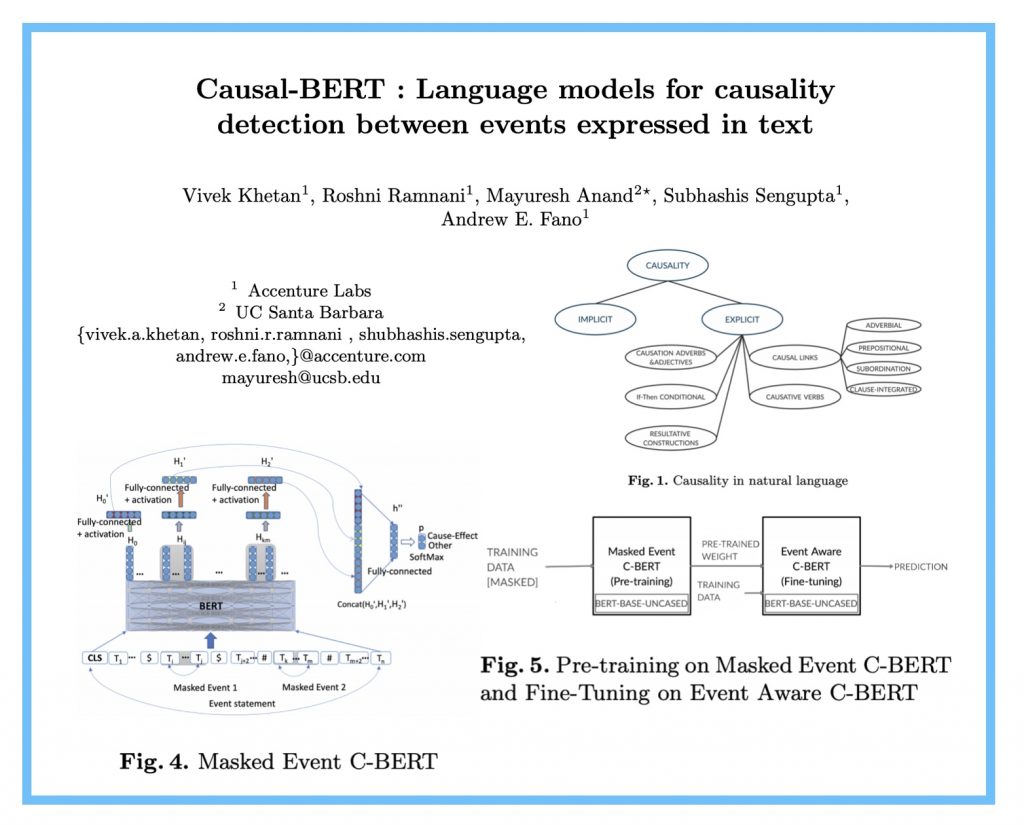
ABSTRACT: “Causality understanding between events is a critical natural language processing task that is helpful in many areas, including health care, business risk management and finance. On close examination, one can find a huge amount of textual content both in the form of formal documents or in content arising from social media like Twitter, dedicated to communicating and exploring various types of causality in the real world. Recognizing these “Cause-Effect” relationships between natural language events continues to remain a challenge simply because it is often expressed implicitly. Implicit causality is hard to detect through most of the techniques employed in literature and can also, at times be perceived as ambiguous or vague. Also, although well-known datasets do exist for this problem, the examples in them are limited in the range and complexity of the causal relationships they depict especially when related to implicit relationships. Most of the contemporary methods are either based on lexico-semantic pattern matching or are feature-driven supervised methods. Therefore, as expected these methods are more geared towards handling explicit causal relationships leading to limited coverage for implicit relationships and are hard to generalize. In this paper, we investigate the language model’s capabilities for causal association among events expressed in natural language text using sentence context combined with event information, and by leveraging masked event context with in-domain and out-of-domain data distribution. Our proposed methods achieve the state-of-art performance in three different data distributions and can be leveraged for extraction of a causal diagram and/or building a chain of events from unstructured text.”
An Interesting Paper — ACCESS HERE