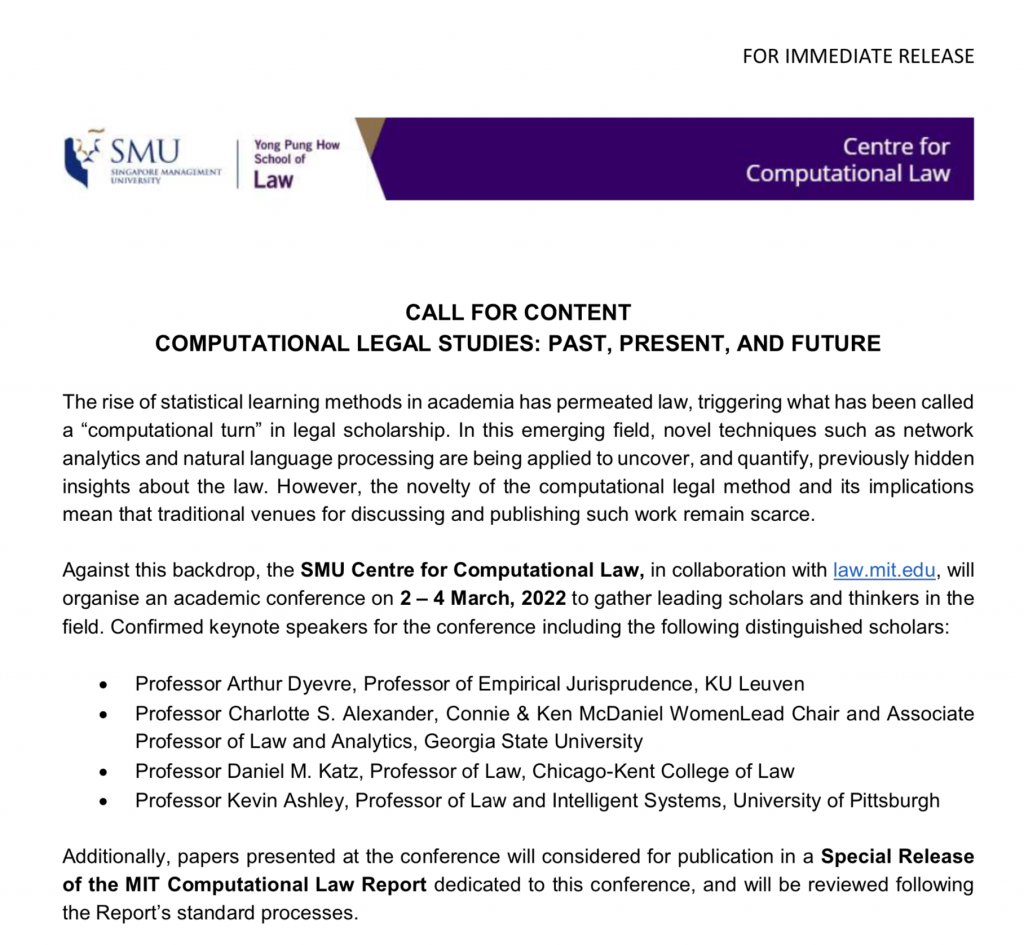
For More Information – please see here (via Jerrold Soh)
Last Night, I delivered a Virtual Talk as part of the Jones Day Visiting Chair of Commercial Law at Singapore Management University — SMU Yong Pung How School of Law.
ICYMI — Michael Bommarito has now become an Active Poster on Linkedin … follow his feed for interesting (and slightly crazy / hilarious / compelling) content !
Read Release from Alan Turing Institute.
Read Underlying Paper in Nature Scientific Reports –
Nadini, M., Alessandretti, L., Di Giacinto, F. et al. Mapping the NFT revolution: market trends, trade networks, and visual features. Sci Rep 11, 20902 (2021). https://doi.org/10.1038/s41598-021-00053-8
Congrats to the team at Burford Capital for ringing the NYSE (delayed due to COVID). Fin Legal Tech is just starting to get cooking … look forward to seeing how it unfolds in the years to come !
It is a great honor to have been selected as the 2021 Jones Day Visiting Professor of Law at Singapore Management University. While my formal in person visit will occur in 2022 (due to delays associated with COVID), I will deliver the Jones Day Lecture via Webinar on November 17, 2021 (9:00am Singapore Time) (FREE ACCESS).
On November 17, I will also join the Honourable Justice Aedit Abdullah (Supreme Court of Singapore) and Mr Mauricio F Paez (Partner, Jones Day) in a panel discussion moderated by Dean Yihan Goh of Yong Pung How Law School Singapore Management University.
**Click Here For Full Programme and Registration**
Click Here For a List of Prior Jones Day Professorship Talks !
Thanks to the Team from CCLA SMU and Dean Yihan Goh for inviting me! I look forward to the Session in November and my in person visit next year.
Law as Code? Code as Law? A long standing debate … but what other ideas / concepts from Computer Science might be leveraged in understanding and managing the law ?
Corinna Coupette, Dirk Hartung, Janis Beckedorf, Maximilian Bother & Daniel Martin Katz, Law Smells – Defining and Detecting Problematic Patterns in Legal Drafting – available at < SSRN > < arXiv >
ABSTRACT – “Building on the computer science concept of code smells, we initiate the study of law smells, i.e., patterns in legal texts that pose threats to the comprehensibility and maintainability of the law. With five intuitive law smells as running examples — namely, duplicated phrase, long element, large reference tree, ambiguous syntax, and natural language obsession — we develop a comprehensive law smell taxonomy. This taxonomy classifies law smells by when they can be detected, which aspects of law they relate to, and how they can be discovered. Our new paper demonstrates how ideas from software engineering can be leveraged to assess and improve the quality of legal code, thus drawing attention to an understudied area in the intersection of law and computer science and highlighting the potential of computational legal drafting.”
It was my pleasure to deliver the (Virtual) Keynote Talk today at LexCon 2021 in Vienna. Next time hopefully in person !
“LexGLUE: A Benchmark Dataset for Legal Language Understanding in English” … Pre-trained Transformers, including BERT (Devlin et al., 2019), GPT-3 (Brown et al., 2020), T5 (Raffel et al., 2020), BART (Lewis et al., 2020), DeBERTa (He et al., 2021) and numerous variants, are currently the state of the art in most natural language processing (NLP) tasks. The question is how to adapt these models to legal text. Inspired by the recent widespread use of the GLUE multi-task benchmark NLP dataset, we introduce LexGLUE, a benchmark dataset to evaluate the performance of NLP methods in legal tasks.
I am proud to have worked with this multi disciplinary research team — who collectively are among the leading NLP + Law Scholars in the world. ( Ilias Chalkidis, Abhik Jana, Dirk Hartung, Michael Bommarito, Ion Androutsopoulos, Daniel Katz, Nikolaos Aletras )
LexGLUE is based on seven English existing legal NLP datasets. However, we anticipate that more datasets, tasks, and languages will be added in later versions of LexGLUE. As more legal NLP datasets become available, we also plan to favor datasets checked thoroughly for validity (scores reflecting real-life performance), annotation quality, statistical power and social bias.
I was happy to provide commentary at the Closing Conference for the project Unlocking the Potential of AI for English Law. It was hosted virtually by the University of Oxford Faculty of Law.