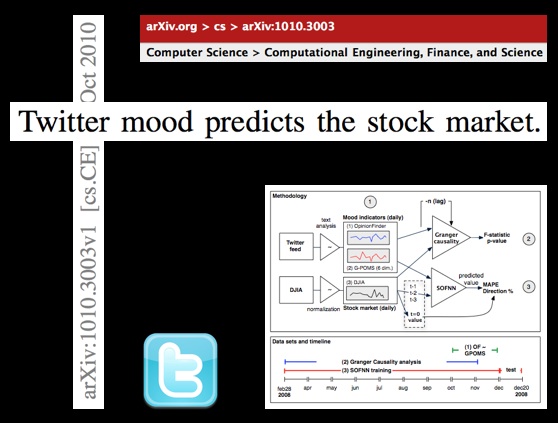
From the physics arXiv comes the interesting paper entitled Twitter mood predicts the stock market. Mike has additional information on the paper over at ETF Central. However — for those who might be interested — here is the abstract:
“Behavioral economics tells us that emotions can profoundly affect individual behavior and decision-making. Does this also apply to societies at large, i.e., can societies experience mood states that affect their collective decision making? By extension is the public mood correlated or even predictive of economic indicators? Here we investigate whether measurements of collective mood states derived from large-scale Twitter feeds are correlated to the value of the Dow Jones Industrial Average (DJIA) over time. We analyze the text content of daily Twitter feeds by two mood tracking tools, namely OpinionFinder that measures positive vs. negative mood and Google-Profile of Mood States (GPOMS) that measures mood in terms of 6 dimensions (Calm, Alert, Sure, Vital, Kind, and Happy). We cross-validate the resulting mood time series by comparing their ability to detect the public’s response to the presidential election and Thanksgiving day in 2008. A Granger causality analysis and a Self-Organizing Fuzzy Neural Network are then used to investigate the hypothesis that public mood states, as measured by the OpinionFinder and GPOMS mood time series, are predictive of changes in DJIA closing values. Our results indicate that the accuracy of DJIA predictions can be significantly improved by the inclusion of specific public mood dimensions but not others. We find an accuracy of 87.6% in predicting the daily up and down changes in the closing values of the DJIA and a reduction of the Mean Average Percentage Error by more than 6%.”