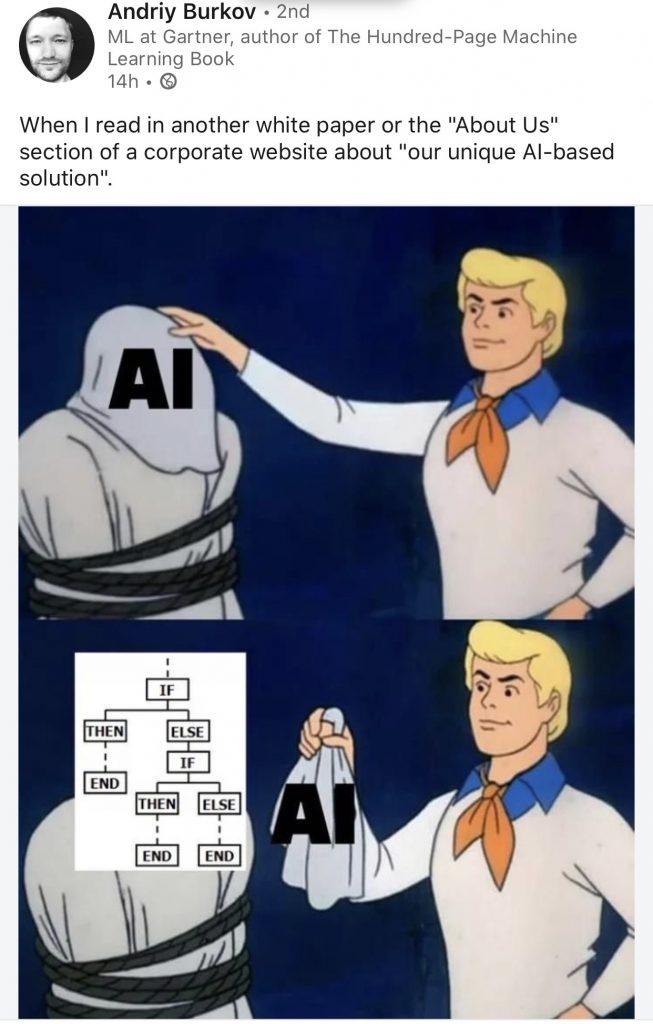
In the broader field of Artificial Intelligence (A.I.) there is a major divide between Data Driven A.I. and Rules Based A.I. Of course, it is possible to combine these approaches but let’s keep it separate and easy for now. Rules Based AI in the form of expert systems peaked in the late 1980’s and culminated in the last AI Winter. Absent a few commercial examples such as TurboTax, the world moved on and Data Driven A.I. took hold.
But here in #LegalTech #LawTech #LegalAI #LegalAcademy – it seems more and more like we have gone ‘Back to the A.I. Future’ (and brought an IF-THEN back in the Delorean). As even in 2020, we see individuals and companies touting themselves for taking us Back to the A.I. Future.
There is nothing wrong with Expert Systems or Rules Based AI per se. In law, the first expert system was created by Richard Susskind and Phillip Capper in the 1980’s. Richard discussed this back at ReInventLaw NYC in 2014. There are a some use cases where Legal Expert Systems (Rules Based AI) are appropriate. For example, it makes the most sense in the A2J context. Indeed, offerings such as A2J Author and Docassemble are good examples. However, for many (most) problems (particularly those with a decent level of complexity) such rule based methods alone are really not appropriate.
Data Science — mostly leveraging methods from Machine Learning (including Deep Learning) as well as Natural Language Processing (NLP) and other computational allied methods (Network Science, etc.) are the modern coin of the realm (both in the commercial and academic spheres).
As the image above highlights, the broader A.I. world faces challenges associated with overhyped AI and faux expertise. #LegalAI also faces the problem of individuals and companies passing themselves off as “cutting edge AI experts” or “offering cutting edge AI products” without an academic record or codebase to their name.
In the academy, we judge scholars on academic papers published in appropriate outlets. In order for someone to be genuinely considered an {A.I. and Law Scholar, Computational Law Expert, NLP and Law Researcher} that scholar should publish papers in technically oriented Peer Reviewed journals (*not* Law Reviews or trade publications alone). In the Engineering or Computer Science side of the equation, it is possible to substitute a codebase (such as a major Python package or contribution) for peer reviewed papers. In order for this field to be taken seriously within the broader academy (particularly by technical inclined faculty), we need more Peer Reviewed Technical Publications and more Codebases. If we do not take ourselves seriously – how can we expect others to do so.
On the commercial side, we need more objectively verifiable technology offerings that are not in line with Andriy Burkov’s picture as shown above … this is one of the reasons that we Open Sourced the core version of ContraxSuite / LexNLP.